A new model from OpenAI, Orion, is expected to be released soon. However, excitement is muted, and expectations for this release are low. There’s a feeling that generative AI is slowing down, and an important person—one responsible for creating ChatGPT—agrees with this argument.
Ilya Sutskever’s perspective. Sutskever, co-founder of OpenAI and a principal architect of ChatGPT, left OpenAI in May to found his own AI startup, Safe Superintelligence Inc (SSI). With SSI, he aims to develop a superintelligence focused on “nuclear-level” safety—using a different path than OpenAI’s current strategy.
Generative AI has stalled. Sutskever told Reuters that the traditional approach of training AI models on vast datasets and scaling up with more GPUs has stalled. He notes that increasing size alone isn’t enough to generate meaningful improvements. Yann LeCun, chief AI scientist at Meta, has also voiced similar concerns.
More isn’t better. Major companies investing in AI continue to deploy more GPUs, which in turn are more powerful and consume more data. However, the progress between successive models has become less noticeable compared to the leaps seen in 2023 and early 2024.
Lots of training for nothing. According to Reuters, labs working on new AI models are grappling with delays and underwhelming results. Training cycles can take months and cost tens of millions of dollars, with no guarantee of success. Companies must complete each cycle before assessing if the investment has led to a performance breakthrough.
Low expectations. Bloomberg recently published another report on OpenAI’s new model, Orion. Despite early buzz, Orion isn’t expected to represent a major leap over GPT-4, with sources close to OpenAI indicating it may take time for the model to be refined. Google’s latest Gemini model reportedly faces similar challenges, while Anthropic has delayed the release of its highly anticipated Claude 3.5 Opus model. Across the industry, new models show only incremental improvements, not enough to warrant an immediate launch.
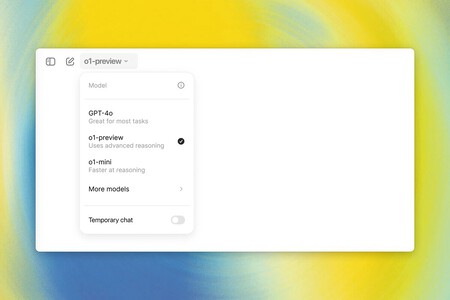
Sutskever remains optimistic. “The 2010s were the age of scaling, now we’re back in the age of wonder and discovery once again. Everyone is looking for the next thing. Scaling the right thing matters more now than ever,” Sutskever told Reuters. The statement is striking but unspecific and doesn’t clarify what he refers to. It’s odd, considering that wonder and discovery accompanied ChatGPT’s early days. Users have become somewhat accustomed to these chatbots, which has diminished that capacity for wonder. For Sutskever, it’s not just about scaling, it’s about “scaling the right thing.”
He has a plan B but gives no hints. The OpenAI co-founder declined to provide details on how he and his team are working to overcome the limitations of current generative AI models. He only hinted that ISS is working on an alternative way to scale training but gave no details. His track record is remarkable, so seeing what kind of solution he brings to his startup will be interesting.
Forcing AI to “reason.” To push beyond current limitations, AI developers are experimenting with a technique known as test-time computing. This method enables models to evaluate multiple answers in real-time, selecting the most accurate response. OpenAI’s model, o1, uses this “reasoning” approach, as do models from companies like Anthropic, xAI, Microsoft, and Google.
From GPUs for training to GPUs for inference. With test-time computing, industry focus is shifting from GPUs used solely for training to GPUs dedicated to inference. Nvidia CEO Jensen Huang recently noted a “second law of scaling,” where demand for inference chips, such as Nvidia’s new Blackwell, is rising rapidly. As these chips roll out in data centers, other companies are expected to compete in the space, seizing the opportunity created by the growing need for inference processing.
Image | Solen Feyissa (Unsplash)